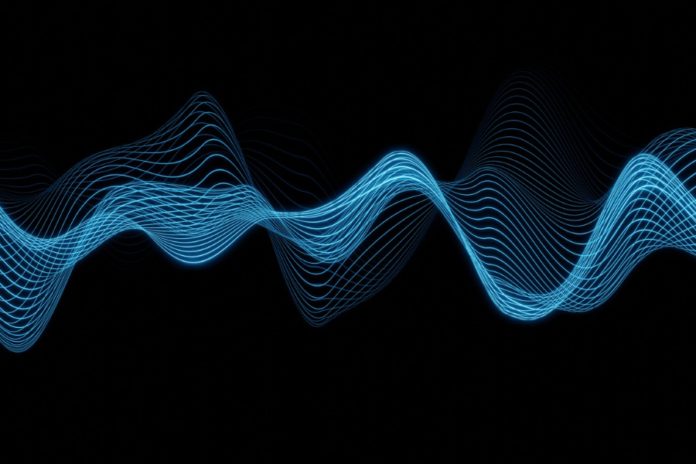
Transcript
Mark:
Welcome to PeopleTech, the podcast of the HCM Technology Report. I’m Mark Feffer. My guest today is Frida Polli the founder and CEO of Pymetrics. They use data based behavioral insights in AI to measure soft skills on the way to creating a more efficient and unbiased hiring process. We’re going to talk about the Pymetrics platform and the science behind it on this addition of PeopleTech.
Frida, thanks for joining me today. I wondered if we could start by having you take us under the hood of your platform. It sounds intimidating, it’s neuroscience based and all that, but what do end users experience?
Frida:
Sure, absolutely. So Pymetrics is a platform that measures people’s soft skills, so cognitive, social and emotional aptitudes that are thought to be things that are more inherent in people. The cognitive science piece, the reason that’s important is, because it allows us to measure these soft skills in people in a different way. So again, my background as I spent 10 years as an academic scientist at Harvard and MIT using all of this technology that we now have productized in the Pymetrics platform for research purposes. So the big aha behind cognitive science was that, you can actually get more objective, better data about people’s soft skills by watching their behavior than by asking them questions. So if you think about traditional soft skill measures, you think about like personality inventories, which are all self report or sort of math tests, which are power tests.
Frida:
They’re all, basically people reporting doing stuff. It’s not so much watching them behave. And we know now from sort of decades of research and actually, sort of looking at the way the world works, that somebody’s behavior is a lot more accurate in terms of predicting who they are than what they say about themselves. And so that’s what we’ve done. That’s what cognitive science has done. It’s created a whole set of activities that people do on the computer.
Frida:
Laypeople like to call them games, but essentially their scientific exercises that people do in like computer that can measure soft skills like planning, or attention, or focus, or decision making, or generosity, or emotional sensitivity in a far more accurate and sort of predictive way There’s all the fancy stuff, but at the end of the day, the basic takeaway message is that, watching people do things is far more accurate and predictive than asking people to tell you about themselves, because people are notoriously bad at knowing themselves and then reporting that in an accurate way.
Mark:
That makes sense. When you started the company, what six years ago, if I’m remembering right. A lot of people sort of approached it as a curiosity, but I don’t sense that’s happening anymore. So what changed?
Frida:
Yeah. Look, I think a lot of things changed. Well, I say a couple things. So one is people realize that there was real science behind this. I think when it first came out, I think people just kind of put it in the bucket of gamified something, something, and it just sounded kind of cheesy. But I think people have realized that, this is an actual scientific discipline that’s been around for decades and is a real alternative to some of these more old fashioned paper and pencil personality quizzes.
Frida:
So that’s one thing. I think, secondly, so where did we learn that behavior is more predictive than asking people questions? It’s actually through technology platforms. So some of the early insights on this were dating platforms. So there’s these great stories of people that go on these dating platforms and they say, I don’t know, it’s a woman.
Frida:
Who’s like, “I want to date a tall, dark handsome guy.” That’s what their profile says. And then meanwhile, they’re messaging, I don’t know, short blonde people, I don’t know, I’m just making this up. And so what they realize is like for whatever reason, people say things about themselves and aren’t true and who knows why that is. We don’t have to be armchair psychologist, but the basic concept here is that actually watching people and observing what they do is just far more accurate. And that helps people right at the end of the day, if you’re self reporting something about yourself and it’s totally wrong, and then you’re making all these decisions based on it, then you end up being unhappy as well as everyone else. So it’s good to get these things right. And so that idea that behavior is predictive, I think is permeated sort of, our understanding of many, many things.
Frida:
And so that’s sort of helped I think the Pymetrics platform. And then last but not least, I think at the end of the day it’s results.
Frida:
I mean, we’ve had so many successful results now with clients who have shown that, yes, not only are they being more efficient, but at the end of the day, they’re hiring people that are better fit to all of the different roles they’re looking to hire into. And oh, by the way, they’re also far more diverse when it comes to gender and ethnicity and socioeconomic status and neuro-diversity. So I think combination of just understanding that it’s a real science, understanding that behavior is really important and also just seeing the successes that our clients have had, have really, I think tip the scale in our favor.
Mark:
Okay. On your website, you say that you work with audited fairness optimized AI.
Frida:
That’s a mouthful.
Mark:
Yeah. What’s it mean?
Frida:
Break it down. Well, basically, so audited just means that someone else has come in and had full access to the platform and said, “yes, Pymetrics does exactly what they say they did. It’s just like an audit. Like you get a tax audit. Somebody comes in and says, “did you fill out your paperwork in the way that you said you filled out your paperwork?” So that’s all that audited means is that we’ve actually allowed someone to come in and do that. And we’re actually the only platform that’s ever done that. And then fairness optimized basically just means that, so when you build an algorithm, an algorithm is a fancy word for an equation. At the end of the day, equations can be… You can tell… Or an algorithm is just a set of instructions. And so I think in the past, the instructions that we’ve given to an equation or an algorithm is just, “Hey, find me a person that’s going to perform best in this role.”
Frida:
That’s great. We should absolutely do that, but what we do is we actually give our algorithms two instructions. We say, find me people that will perform really well in this role and do that without bias. So we are sort of dual optimized or we have two sets of instructions that we give all of our algorithms to not only find the best suited people, but to do it in a way that is unbiased from a gender and ethnic perspective. Does that make sense?
Mark:
Yeah.
Frida:
That’s really what it means is that we’re not sacrificing either instruction set that we’ve given, we’re actually optimizing for both.
Mark:
Now, how unique is your whole approach and your technology? Are there other companies out there who are even close to being in the same box?
Frida:
Yeah. Look, some of the things we do are not that unique. So other platforms that I know also have this sort of dual will set of instructions, which is like definitely focus on prediction, but then also definitely focus on being unbiased. Another platform called Hired Score does this as well. That’s one that I know their technology well, and I’m confident and there are probably others. I don’t actually know. It’s one of those things that I think in the field, there’s a lot of marketing buzzword and people say they’re ethical and they’re fair, but who knows what that actually means? It’s sort of like when people would talk about healthy foods, well, what does that even mean? So I know of a few platforms that do this, but I would also say that, a whole host of other platforms don’t actually do this.
Frida:
They’re not being as sort of curious and thoughtful and methodical about looking at all of the inputs that go into the algorithms and saying, “Hey, are they helping the bias issue or are they actually hurting it?” And that’s really sort of fundamental for our platform is really ensuring that we do that and we take a very methodical approach. And so again, it’s hard to answer that question, Mark. I don’t know of anyone one that’s really come out and said, “yes, we do exactly this. We look at all of our algorithms, we ensure that there is no bias in them.” You see a lot of buzzwords being thrown around like fair, but fair actually has so many different meanings that it doesn’t ultimately mean anything. The fact that we are unbiased is actually something I don’t see a lot of vendors saying in part, because it’s pretty hard to actually achieve.
Mark:
Now let me ask you one more question about, about the platform.
Frida:
Yeah.
Mark:
You talk a lot about transparency and you used the phrase transparent technology, which I thought was kind of cool. Can you tell me about that? What’s the thinking behind it and what’s the purpose?
Frida:
Sure. Yeah. So, I mean, it’s back to sort of, why would we do an audit? We actually had a group of academic researchers come in and we gave them full access to the platform and they got to look at the data. They got to look at code, they got to look at everything. Well, why would we do that? It’s all about transparency. It’s one thing to say, “I do something,” it’s one thing to say, “oh, all this great stuff that I just told you, I optimize for lack of bias, blah, blah, blah.” But if no one has been able to come in and shine a light on that technology and say, “are they actually doing what they say they do?” Then it lacks transparency. And how can we actually believe it? Does that make sense? So I think that transparency is so important when you’re trying to build trust.
Frida:
It’s not the only thing I’m not saying like, “Hey, that’s the be all and end all.” But I think it’s a really important aspect. And I mean, we use sort of analogy of food labels. If you’re trying to say you’re a diabetic and you’re trying to reduce sugar intake and every single food item that you put in your mouth had no product labeling on it. My goodness, you could die of an insulin shock, if you know what I mean? Because you’d have no idea like candy bar versus broccoli sprout. I mean, I don’t know if you had no labels and you’d never been taught, you could eat one or the other and not know any different. And so that’s really what it is. It’s just providing labeling to the technology. And also, again, these nutritional labels that are on food products have an outside agency that comes in and says, yes, that’s actually true.
Frida:
It’s not the manufacturer of the food gets to put whatever label they want. And so it’s really that dual process of having some sort of third party verification, as well as having some labeling factors that you’re putting on your tech that I think is super important. And I mean, just on this note, there was a piece in the New York times today talking about a group of companies that have come together to create a bias auditing framework. Yesterday, the World Economic Forum came out with a similar type of guide.
Frida:
So I think increasingly we’re going to see these audit frameworks put out by whether it’s a private group of companies or whether it’s sort of more public entity like the World Economic Forum. But the point is I think that something consumers really want to know about these tools. At the end of the day, why are people afraid of algorithms? They’re afraid of algorithms in large part, because a lot of what you read in the news you is, “oh my gosh, Amazon had a biased resume pasture, or facial analysis is biased.”
Frida:
It’s not so much that… That’s really the thing that people are the most afraid of with good reason. We don’t want to be sort of engaging with these bias systems. And so we think that the more we can do to be transparent about lots of things about our platform and bias is just one of those things. Another way that we’re transparent is that once someone goes through Pymetrics, every single person gets some information about themselves as we call it a development report. So our whole point is like, “Hey, let us tell you what we measured and let us tell you who you are.” Now again, it’s not like the perfect system. I’m sure there’s other ways we could do it, blah, blah, blah. But it’s being transparent about what we’ve done, what we’ve learned and hopefully giving that information back to the consumer. So I think it’s treating consumers with respect and treating them like you, or I would want to be treated, which is, I want to know as much as I can about this process that you’re putting me through.
Mark:
Okay. One more question.
Frida:
Sure.
Mark:
We’re at the turn of the year.
Frida:
Yeah.
Mark:
So what do you foresee for Pymetrics in 2022?
Frida:
I think Pymetrics is at a really incredibly cool time and its history, because we started out in talent acquisition and that’s when people know us for like, “oh, recruiting games, blah, blah, blah, campus hiring, blah, blah, blah.” But we’ve moved so much further beyond that to essentially being a soft skill data layer that really can be introduced anywhere. And so I think, I mentioned this to you earlier, we’re doing a lot of work with educational platforms to see, “Hey, can we personalize learning, using soft skills.” We’re doing work with sort of retraining and re-skilling platforms to say, “Hey, can we point people in the right direction if you’re going to spend six months, 12 months of your life, skilling up for a new career, can we help you understand what that is?”
Frida:
Can we just provide insights to CHROs about what are the soft skills that make their entire workforce unique? And then how does that differ by role by geography, by tenure level and so on and so forth. So it’s really being that radar screen into the soft skills of your company, which right now we have no information about right, like absolutely zero. And being that information layer about soft skills broadly, not just in this very narrow field of talent acquisition, but in talent mobility, in re-skilling, in learning and development, in educational context. And that’s what’s so exciting to me, because at the end of the day, I think this layer of information can, and is so helpful in so many places. And that’s really where we want to see the platform expand.
Mark:
Frida to thank you very much.
Frida:
Absolutely. Thank you so much, Mark.
Mark:
My guest today has been Frida Polli, the founder and CEO of Pymetrics. And this has been people tech, the podcast asked of the HCM Technology Report. We’re a publication of recruiting daily.
We’re also a part of Evergreen podcasts to see all of their programs visit www.evergreenpodcasts.com.
And to keep up with HR technology, visit the HCM technology report every day. We’re the most trusted source of news in the HR tech industry. Find us at www.hcmtechnologyreport.com. I’m Mark Feffer.
Image: iStock