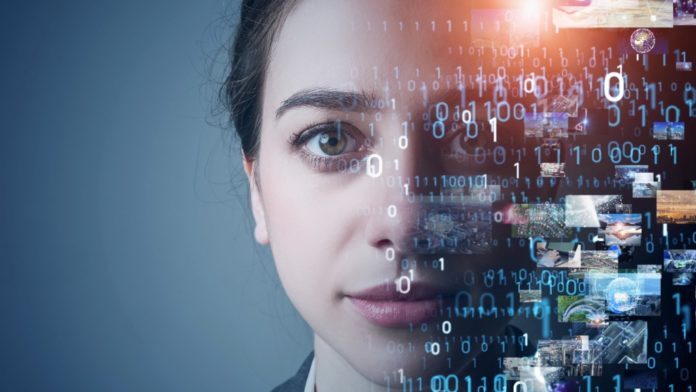
Mark (00:00):
Welcome to PeopleTech, the podcast of the HCM Technology Report. I’m Mark Feffer. Today, I’m talking with Eric Sydell and Mike Hudy. They’re the executive vice president and chief science officer for Modern Hire. We’re going to talk about analytics and bias, AI, of course, and their new book called Decoding Talent. And we’re going to do it all on this edition of PeopleTech. Hey Eric. Hey Mike. Welcome. So, tell me about your book, what’s it about, but also what spurred you to write it?
Eric (00:47):
Yeah, it’s a great question. Obviously we all know that AI and big data are all the rage are having a huge impact on the world, in every technical domain that there is, and also in HR and in talent management, for sure. And we spend a lot of our time analyzing and understanding candidate data and helping our clients to figure out what to do with that information, how to use it in the hiring decision to make the decision better and more effective and predictive and valid and fair as well. And so that being the lions share of what we think about and do for the last two decades sort of has led us to the ideas that we wrote about in Decoding Talent, where we really talk about how to apply that model and that idea to HR and to talent management decisions in general.
Eric (01:47):
And you know, of course HR, the practice of HR goes back more than a 100 years, but only in the last few decades has HR begun to be a real strategic business partner. And now with AI and with big data, there is just tremendous possibility for what HR and talent management organizations can do. And we wanted to communicate that vision and help explain to people what we see from living in this world every day and the power of these techniques and what they can do to make decisions better and more fair, how to explain that to HR organizations and talent managers from a high level to help them see what’s possible and hopefully start taking steps to take real advantage of some of these modern tools.
Mark (02:42):
And Mike, did you have anything to add on that?
Mike (02:45):
Yeah, just to add to that. I mean, Eric and I, we spend a lot of time educating the market, we do a client by client because there’s not a great understanding of AI and there’s a universal fear of it, and there’s a lot of stories out there that emphasize the negative and certainly AI has to be well developed, implemented right, tested, but we have a chance here, Eric and I, and Modern Hire we’ve been doing this for a long time. We’ve been doing, putting in database objective solutions with clients for over two decades, and we’ve seen the power of it, we’ve seen the power of how well it works, how it helps companies do a better job of hiring, doing a better job of getting it right, getting people who actually stay, enjoy themselves, perform well, but also driving more diverse workforces too.
Mike (03:37):
So, this was an opportunity, another vehicle to share our insights, share our learnings in a very as you said before, we got on in a way that’s very understandable non threat to demystify AI a bit, just to say it’s really about just using data, making more objective decisions and learning from what we’ve done in the past. And AI is just a vehicle, a statistical technique that allows us to do that on a different scale and to make sense of data that might not be structured beautifully, that’s quite unstructured and messy.
Eric (04:14):
Yeah. And that rings a bell like with me too, the other day I was mailing a copy of the book and the person behind the counter saw the title of the book and she said, “I’m scared of that stuff.” And I said, “What stuff? Like what do you mean?” And she said, “Robots and stuff, and AI.” And I said, “Well, okay. I mean, that’s not what the book is about.” So, to Mike’s point, there’s a real need to demystify these technologies, we’re not talking about Terminator robots when we talk about AI, we’re talking about statistical tools that help us understand data.
Mike (04:51):
And I think another, in the book we go through a lot of examples, a lot of real world examples, specific cases, and then more broad cases with clients. But what we’ve seen is that humans aren’t great at making decisions, so there’s a lot of talk about AI and the potential for bias with AI, and we talk about how to do things right in the book, but what’s not talked about as much is what is the danger of not using data and objectivity in decisions, and it’s where we are today. Like the reason we have institutional bias built in and if you look across the world of work and the opportunity to work and management levels, when you look at the diversity or lack thereof, that’s a result of humans making decisions in using flawed techniques for decades and decades and AI offers the promise of helping make that right.
Mark (05:49):
Now, analytics has been sort of gaining traction for lack of a better term, it’s moving steadily into HR and talent acquisition. Generally are employers getting as much out of it as they can, are they educated properly? Are they willing to be educated properly?
Eric (06:12):
Well, I think absolutely they are not yet getting everything out of analytics that they can. I mean, that’s, I think that’s a long road, and a lot of what we talk about in the book is that you’ve got to get your data house in order. There’s a lot of difficulty that companies have analyzing data, and it’s funny because we live in, obviously we know we live in a big data world, there’s tons of data, there’s tons of information being collected all the time and produced and so forth and et cetera. But the problem is most of that information is not in a connected analyzable format, and so we have situations where a client will ask us to analyze, for example, resume data, and then come back to us a week later and say, “Nevermind, because we can’t figure out how to get the resume data out of the database that it’s stored in.”
Eric (07:03):
So, they’re storing the data, but they can’t analyze the data, so I think that is a very common refrain in organizations, tons of data, but not necessarily access to that data. You know people store data for record keeping purposes, for compliance purposes, in case they ever have to look something up, they historically haven’t stored it to analyze it and that’s a different thing. So, I think that’s the first step in all of this is really getting your data house in order, figuring out what data you’ve got, what you can get access to, how you can store it in a way that you can analyze it, and also do that in a way that respects privacy boundaries and laws and things, because those are very prominent issues now as well.
Mark (07:48):
And Mike.
Mike (07:49):
And I agree with Eric and the way we look at it is sort of walk before you run to get to your destination, even if you’re walking, you’re at least making progress towards it you’re not going to get there all at once, and there are wins to be had just by and Eric reference to the six step process we talked about putting in place where it just starts with getting your house in order, getting your data in order, your databases, figuring out how to connect them and you’re not going to have it all figured out instantly, but when you start to bite, take off bite size chunks, it can be helpful and it makes me think of one of the, I think it’s actually an example cited in the book there’s value to learning from data even to educate yourself on what not to do and things to stop doing because the data doesn’t support it.
Mike (08:36):
And we had a huge multinational organization that was recruiting store managers to run their stores, and they ask a bunch of pre-qualification questions in their application process. And these were developed in the way that most are developed, you have recruiters, you have hiring managers and then we say, “Okay, what are the requirements? What experience are we looking for people we want to come in and run our stores?” And so they came up with this list, “Well, they have to have X amount experience, they need this amount of experience working in restaurant.” So, they create all these minimums and these become knockout criteria on the front end of the process, but what very few organizations do is track that data and then look at it over time to see, does it tell us anything about how good our store managers are and learn from that data.
Mike (09:37):
And so we worked with this particular client and they had well over 50 of these pre qualifying questions that were being used in different markets and we took the 50 most popular and took then data on, did these store managers that were ultimately hired to actually stay, and how were they performing? And we found that of the 50, only five of them actually linked to success on the job. So, there were 45 questions being asked that had no relationship whatsoever to your success, but there were candidates in the pipeline being knocked out because they didn’t have a certain amount of experience. So, for instance, the restaurant experience one, we found actual inverse relationship, the more of it you had, you actually did worse and they had been using it to screen out people if you didn’t have enough.
Mike (10:27):
So, there’s value to even having that in the database and then, okay, that lives in your applicant tracking database. Now, I need to connect that to actual store metrics and how these people are performing, which doesn’t happen automatically so they basically, we work with them to do that hard work. Now you have the two connected, now you’re in business, you can start to look at data, it’s not the entire picture, but it certainly helps you the first thing you ask are these qualifying questions, if they’re bad, that has huge implications and so their based on that analysis, they change their pre qualifying questions.
Mark (11:02):
Could we talk a little bit about analytics and bias? You mentioned it before Mike, it seems like the discussion is deeper or should be deeper than a lot of people realize. Because it’s not just about looking at analysis and then making a bias decision based on it, there’s more to it than that. Could you talk about that?
Eric (11:30):
Yeah, I can. I’ll start bias is one of the biggest, I think and most interesting topics of our time because of the influence of big data and AI a few years ago, everybody started applying AI, which is very powerful to let sets of data, to come up with predictive tools and algorithms and things and they trained the AI on these big sets of data. And then they would have these AI algorithms that they would use to scale up some sort of process to decide who gets credit for a house or for who gets a job or who gets all these different things, right. But in, so doing, they were basically amplifying the bias that was in that set of data across this entire population. And that is very bad obviously, and I think a lot of organizations are learning to do it better now and in a more careful way.
Eric (12:27):
But the thing that’s so interesting about that is that while in those early examples, AI was magnifying existing bias. If you think about it’s also identifying the bias I mean, it is so powerful that it’s allowing us to see inside these huge sets of data and understand where bias is in a way that we couldn’t before. And so to me, like the exciting part is, okay, we can use AI to identify bias and guess what, if we can identify it, we can also get rid of it. So, that’s the challenge, but it’s anytime you’re dealing with a huge set of data, there’s likely many layers of bias built into that data, I think of bias is sort of an onion you peel back one layer and you find another and you keep looking at it and you keep peeling it back, we can never stop doing that, and we’re never going to solve bias because it’s inherently a part of everything.
Eric (13:23):
So, I think that people, a lot of times people equate the word bias with like racism or something and that’s not right. We have a million cognitive biases in our human brains that affect the way we process information, so this is just the process of getting those out of the way of finding them and getting them out of the way so that we can make better, more objective, fair decisions. And AI’s hugely powerful and helpful in that as long as it’s done in the right way.
Mark (13:51):
Mike, did you have anything to add for that?
Mike (13:53):
Yeah, I think obviously it’s a hot topic and rightly so, but I think the way that it’s discussed not overtly, but the way that I read it is there’s this assumption that there’s no bias today and then we introduce these techniques and my goodness, there’s bias. I think what we all need to acknowledge is there’s huge bias today with the broken traditional ways that we’ve been doing things which is looking at resumes and applications, doing manual interviews, and as Eric said, all of these cognitive biases that we have, that we can’t avoid as humans, we can be trained, but we can’t train them all out of us it’s where it’s gotten us to where we are today. So, data, AI, making more objective decisions based on data offers the promise to help mitigate that.
Mike (14:47):
We can, it’s easy to train AI to say, “Don’t look at the facial, we don’t care.” Or to hide any information about that and not even show the AI of video image or audio image and only train… So, there’s things that we can do that as humans, you can’t tell people systematically across hundreds and hundreds of recruiters, don’t do this, don’t look at this, don’t look at what the candidate’s wearing, don’t look at the background, don’t overrate them because they’re very similar to you, those are all things that we know we do as humans. It’s very easy to train the AI to do that less so with humans, so I think that’s where the conversation has to start and we’ve seen evidence of that when we look at our own data, we have these big clients that do high volume hiring, that we’re hiring with traditional techniques before they started taking a more data oriented approach.
Mike (15:37):
And we start to see the diversity of their higher pool start to change when you’re looking at data, when you’re looking at the job relevant factors and the machine is able to screen out the things you shouldn’t be thinking about, and you start to see a more diverse pool of candidates coming in.
Mark (16:01):
Now, your book, I don’t want to say it’s deep in a bad way. I mean, but it’s about data, it’s about a lot of fairly sophisticated concepts and I was wondering as I read it, is this the kind of thing that you share with your customers when you’re advising them and how do they react to it?
Eric (16:27):
Yeah, I mean the book is it’s positioned as sort of a vision of the future, it’s taking our sort of insights that we’ve collected over the years and trying to package them in a way to communicate what we see as such an amazing future possibility for HR and talent management. I think what we do as Modern Hire fits into that, but it’s not like the book doesn’t map 100% to the products and services that we offer today, it’s a future vision and it’s us trying to educate the market and push it along to adopt some of these technologies. We certainly do a lot of things that fit into the mentality of the book and the philosophies that are in the book, but it’s not a one-to-one relationship and so I think clients in my experience have appreciated it, have gotten some insight out of it and have learned to see some of the possibilities that they hadn’t seen before, and Mike May have some additional insight there.
Mike (17:32):
Yeah. I don’t think, we don’t come at it this way necessarily with our clients, but I almost I see this as us sitting back and like looking at this is the collective wisdom that we’ve gained through the past 20 years and AI has really exploded, but in the last several years, but this approach, and we introduced that six step model that’s what we’ve been doing even before we were using machine learning or deep learning, it still holds true that you need to get data, you need to connect the data sources, you need to analyze that data, see what it tells you and then based on that, build a scoring algorithm to model that, and then refine over time. We did that with regression 15 years ago, same kind of thing, so the thinking, and that’s what we’re trying to do is like, let’s not get hung up necessarily on AI, AI is super powerful, but the fundamentals of like, let’s look at data, let’s analyze data and model it have always been around and have that informed by theory and best practice.
Mike (18:33):
But now AI just opens up a whole new realm where the way that we can model data is so much more sophisticated, and it’s a good thing because we can take messy data, like open ended content, no longer multiple choice, and be able to model that. So, it’s really about trying to, like we don’t get into the, it’s not technical from a, we’re not talking about here’s our preferred approach for doing machine learning, or here’s why deep learning offers huge advantages. It’s like, you need technical people to figure that out, but first we need HR people who are the decision makers to embrace this whole concept and say, “Yeah, we need to bring this to our organization and we can go out and get vendors, or we can go out and hire people, we probably already have people sitting in our organization.” Which we’ve seen more and more, you have more and more data scientists, getting involved in HR.
Mike (19:25):
So that’s really the… We’re taking something very technical, we’re leaving the technical aspect of it behind, but more embrace the concept of where this is going, because it’s the way the future in terms of hiring and talent management in general.
Mark (19:39):
Well, that’s sort of a holy grail, isn’t it? Sort of eliminating the technical speak from the use of data and analytics so that people use it as an appliance.
Mike (19:55):
Yeah. I mean, that’s really it because HR as a field, it’s changing, but HR as a field you look at the background of people who end up in HR, they’re typically not your stats gurus, they’re not somebody who went to schooling and got a stats degree. And so this can seem threatening, but you don’t need the HR people to be doing this, there are specialists and there’s lots of them out there that can do it but what you need is you need HR and HR is the guidance, they’re the ones that can take a data scientist and say, “Hey, we can’t look at these factors because we know legally we can’t.” So, they’re the ones, they bring an expertise to the data scientist that the data scientist isn’t going to have, and you put those two things together, like AI harness the right way, guided by somebody who knows the ins and outs in HR and the regulations around hiring then you have something.
Mark (20:53):
You begin chapter two by talking about Star Trek, as an analogy for management, basically. And you’re talking about chain of command and so I’ve got to ask what’s the better management model Starfleet or Klingon?
Eric (21:16):
I’m not sure I guess, I mean, maybe Starfleet I hope, I mean, seems less scary at least, right?
Mark (21:24):
Yeah, absolutely. Mike, just, do you want to go on record?
Mike (21:29):
Full disclosure never watched Star Trek, so I’m not in a position. Our third author, I believe is the Treky that helped us come up with that example, so.
Mark (21:42):
Eric and Mike, thanks for coming by this morning, it’s been great to talk to you.
Eric (21:46):
You too. Thanks a lot. Appreciate the time Mark.
Mike (21:49):
Thanks Mark. Good talking to you.
Mark (22:00):
Our guests today have been Eric Sydell and Mike Hudy of Modern Hire. And this has been PeopleTech, the podcast of the HCM Technology Report. We’re a publication of RecruitingDaily, we’re also a part of Evergreen Podcasts to see all of their programs visit www.evergreenpodcasts.com. And to keep up with HR technology, visit the HCM Technology Report every day with the most trusted source of news in the HR tech industry, find us @www.hcmtechnologyreport.com. I’m Mark Feffer.
Image: iStock